Top 10 AI Inference Startups And What Sets Them Apart
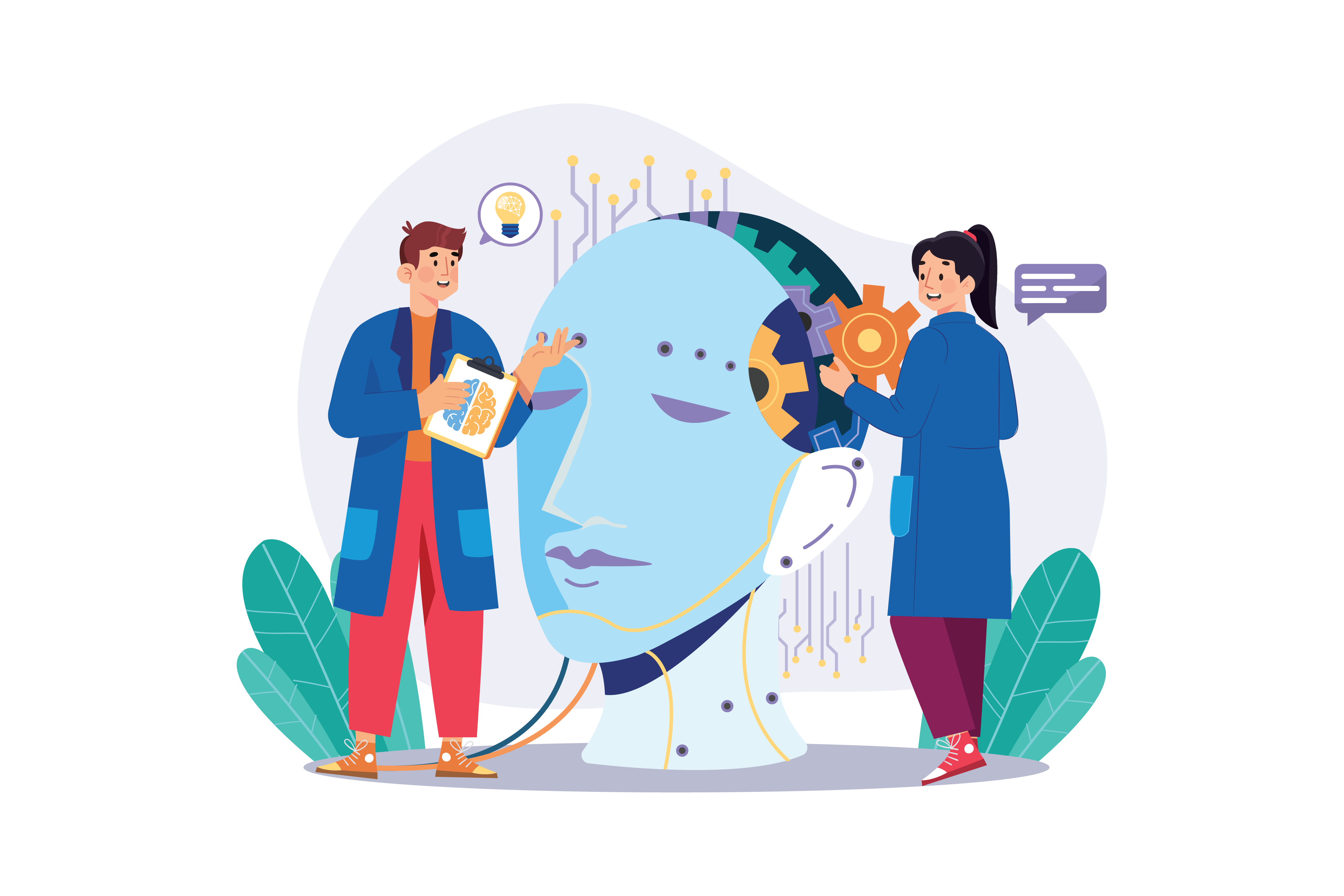
Understanding AI Inference
AI inference is where the magic of machine learning truly comes to life. After a model has been meticulously trained on vast amounts of data, uncovering intricate patterns and encoding them into its parameters, inference is the moment it steps into the real world. This is the phase where a model transforms from a sophisticated algorithm into a decision-making powerhouse, making predictions and driving actions based on entirely new and unseen data. It's the ultimate showcase of the model's learned intelligence, bringing cutting-edge solutions to real-world challenges.
How It Works

In the training phase, neural networks learn by analyzing labeled data, uncovering patterns, and fine-tuning their ability to make accurate predictions. This stage is resource-intensive, requiring significant computational power to optimize the model. Once trained, the model moves to the inference phase, applying its learning to new data and generating outputs like classifications, translations, or predictions. Unlike training, inference is designed to be fast and efficient, enabling seamless real-world applications that demand quick and reliable results.
The Advantages Of AI Inference
AI inference offers numerous advantages, enhancing decision-making and automating tasks across various industries.
- Real-time decision-making enables instant responses to complex queries, as seen in chatbots that utilize real-time inference to answer user questions immediately.
- Personalization is another key benefit, with AI inference allowing for the dynamic customization of content and services for individual users.
- Optimization of workflows in real-time by AI inference drives operational efficiency.
- Cost efficiency is also achieved by streamlining inference tasks, making them less expensive than the costs associated with training AI models.
Innovators are creating cutting-edge hardware and software to make AI smarter, faster, and more accessible across industries. So, of course, we have service providers focusing primarily on this aspect of AI workflows. And enter AI inference startups into the scene. Whether it's optimizing chips for edge devices or streamlining cloud deployments, these companies are transforming how AI operates in the real world.
Now, let’s take a closer look at some of the top AI Inference startups functional in today’s market.
Top 10 AI Inference Startups
1. Anyscale
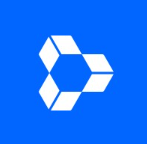
Founded: 2023
Focus: Anyscale offers an AI platform that features cutting-edge tools and modular components to optimize AI workload performance and reduce costs. It allows developers to construct and scale AI applications utilizing Ray, a Pythonic API, for distributed computing that spans CPUs and GPUs, delivering fault tolerance, reliability, and precise orchestration features.
2. Together AI
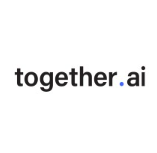
Founded: 2022
Focus: Develop AI acceleration solutions for edge computing applications. Their platform, Metis, combines hardware and software to handle computer vision inference at the edge, making it suitable for real-time applications in various industries.
3. Baseten
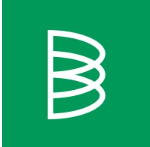
Founded: 2019
Focus: Baseten offers a platform to help companies with high-performance, secure, and dependable model inference services, reducing the time and effort required to go from concept to deployment. It enables teams to focus on creating the best possible AI products without worrying about ML infrastructure.
4. Replicate
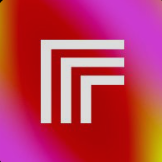
Founded: 2019
Focus: Replicate allows one to run and fine-tune AI models with an API and deploy custom models at scale, all with one line of code. It provides access to thousands of models contributed by the community that actually work and have production-ready APIs.
5. Initializ
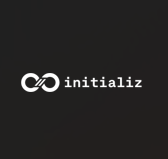
Founded: 2022
Focus: Initializ simplifies scalable, secure AI inference with support for Llama 3 and Whisper models. It enables quick deployments, cost optimization, and streamlined development with automated GitOps and CI/CD, reducing failures and accelerating delivery.
6. Fireworks AI

Founded: 2019
Focus: Firework AI develops purpose-built AI computing systems explicitly designed for the complexities of AI inference applications. Their architecture enables scalable real-life AI applications, enhancing performance and efficiency.
7. Modal AI

Founded: 2021
Focus: Modal provides serverless cloud infrastructure for AI, machine learning, and data applications, allowing developers to define hardware and container requirements next to their Python functions. It is designed to scale to hundreds of GPUs in seconds and offers sub-second container starts.
8. Deepinfra
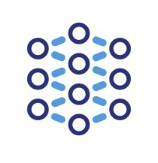
Founded: 2022
Focus: Deepinfra provides fast, low-cost, scalable, and production-ready infrastructure to run top AI models using a simple API. It allows one to deploy models to production faster and more cheaply than developing the infrastructure, with serverless GPUs and pay-per-use pricing.
9. CentML
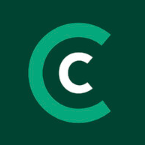
Founded: 2022
Focus: CentML allows one to effortlessly train, fine-tune, and infer AI models while optimizing hardware infrastructure. It cuts down LLM deployment time from weeks to minutes and saves costs with more efficient hardware utilization.
10. Groq

Founded: 2016
Focus: Groq provides fast AI inference for openly available models like Llama 3. One can move seamlessly to Groq from other providers by changing three lines of code, and independent benchmarks prove that Groq Speed is instant for foundational openly available models.
Wonder What Makes Them Better Than The Others?
When comparing AI inference startups, several key metrics are crucial for evaluating their performance and capabilities. Here are the primary metrics used in this analysis:
- Latency: AI response latency is the time between receiving input and generating output. Low latency is vital for real-time applications like autonomous vehicles and voice assistants. Key components include Time to First Token (TTFT), the initial delay, and Total Response Time (TRT), the time to complete the output. Reducing latency ensures faster and safer AI interactions.
- Throughput: Throughput measures how many requests or tokens an AI model can process per second. It's crucial for handling large data volumes in chatbots, real-time analytics, or content generation applications. High throughput ensures scalable, consistent performance under heavy workloads.
- Model Size and Memory Requirements: AI model parameters and memory needs impact deployment on resource-limited devices like smartphones and IoT systems. Larger models offer better accuracy but require more resources. Balancing size and efficiency ensures seamless, accessible AI performance across platforms.
Basically, the next time you look for optimisation of AI inference for your own product, do keep an eye out for these details. And head over to our blog repository for more information!